If you are following my blog, then you might be familiar with ANN till now. Still, let us define Artificial Neural Networks as the powerful and a very strong tool which mimics the human brain.
As our brain has neurons which help us send and receive signals through Axons and Synapses, similarly machines are being developed to act like a human being. Although it could not enact the same, it is pretty well.
Let’s dive into our today’s topic, Activation Functions.
What is an Activation Function?
Activation Functions are the functions which when introduced to the input, brings out Non-linear properties to the network. The main purpose of an activation function is to generate output from the input.
In ANN we perform the sum of products of inputs(X) and their corresponding Weights(W) and apply an Activation function f(x) to it to get the output of that layer and feed it as an input to the next layer.
What if we do not activate the input signal?
If we do not apply the Activation function, then the output signal will only be a Linear function. Although a linear function is easy to solve, it will possess less functional complexity and mapping.
We want our Neural Network for linear functions, we want it for more complex functionalities. Also without activation function, our Neural network would not be able to learn and model other complicated data such as images, videos, audios, speech etc. That is why we use Artificial Neural network techniques (Deep learning) to make sense of something complicated, high dimensional, non-linear-big datasets, where the model has lots and lots of hidden layers in between and has a very complicated architecture which helps us to make sense and extract knowledge from such complicated big datasets.
Why do we need Non – linearities?
It is something interesting if it stuck to your mind. If the function is a linear one, then it will not have those non-linear properties that are needed to understand the model in a more deeper manner. If we want to identify, which one is linear and which one is non-linear, then there is a way. If there is a curvature on plotting the function, then it is non-linear.
You will be glad to know that Neural Networks are considered as Universal Function Approximators i.e. neural networks can compute any function.
Hence, we need the Activation function to make the Neural networks more powerful and to learn something complex.
Activation functions are always differentiable.
To activate means if the particular neuron is acceptable to the network or not i.e. if we are giving any neuron, then there is a particular range in which it appears. If that range is suitable for the network, it is activated else, not.
On this concept let’s discuss various types of activation functions:
Variants of an Activation function
-
Step Function
Now, if we talk of a range, then the first thought that comes to our mind is setting a threshold value above which the neuron is activated and if the value is below the threshold value, then it’s not activated. Sounds easy. Yes! It is. This function is called as Step Function.
See the figure below to understand more:
Output is 1 i.e. activated when value > 0 and 0 i.e. not activated when value < 0.
Those things which seem easy carries many drawbacks with them. So is the case here. This function is applicable only there where we have only two classes. Hence, for more classifications, we can’t use this. Moreover, the gradient of the step function is zero, i.e. during propagation when we will calculate the error and send it back for the improvement of the model, it will reduce it to zero which will stop the improvement of the model. Hence, the best possible model will not come out.
-
Linear Function
We saw the problem of gradient being zero in step function. This problem can be overcome by using the linear function.
f(x) = ax
If we take a = 4.

Here, the activation is proportional to the input. One of the problems of only 2 classes has been overcome here as by putting any value to a, x can correspond any value and many neurons can be activated at the same time using it.
Still, we have an issue here. If we take the derivative of this function. Then it comes out to be:
f'(x) = a
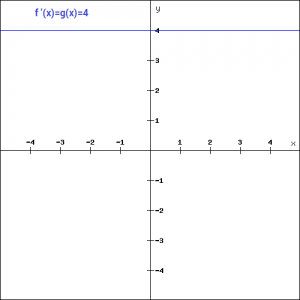
The derivative corresponding to the linear function is always a constant and does not depend on the input given. Hence, whenever we find the gradient it comes out to be 4 in this case. Hence, we are not really improving the model. We are just giving a fixed value. Also, suppose we have multiple layers and transformation is needed to be done in each layer, then the output comes out to be the linear transformation of the input given. That means, instead of multiple layers we could have one single layer. No matter how we are putting, the whole network still acts as a single layer. Hence, linear functions are good for simple tasks where interpretability is needed.
Linear functions are used only at output layers.
-
Sigmoid Function
It is one of the most widely used activation functions. It is a non-linear, ‘S’ shaped curve. It is of the form:
f(x)=1/(1+e^-x)
The X values range in -2 to 2. Notice that the small changes in x produce large changes in Y, i.e. Y is steep. The range of the function is 0 to 1.
It is a smooth function and is continuously differentiable. the main advantage it possesses over step function and the linear function is that it is non-linear.
If we look at it’s derivative:

If we look in the above graph then we find that the curve is smooth and dependent on x i.e. if we calculate the error, then we will get some value to be used for improving the model. We can see that beyond -3 to +3, the function is almost flattening, i.e. it is approaching to zero which indicates that the error will now be minimal and the model will stop learning. This problem is termed as ‘Vanishing Gradient‘.
One more problem that sigmoid function offers is that its range is between 0 and 1 i.e. it is not zero centered i.e. it is not symmetric about the origin and also all the values are positive.
It is usually used in the output layer of binary classification, where results correspond to either 0 or 1.
-
Tanh Function
The tanh function is almost similar to the sigmoid function. It is actually the scaled version of the sigmoid function.
tanh(x)=2sigmoid(2x)-1
or it can be written as:
tanh(x)=2/(1+e^(-2x)) -1
The tanh function is symmetric over the origin.

Its range is between -1 to 1. Hence, it solves the problem of the values being of the same sign. Its optimization is easier hence, it is always preferred over Sigmoid function. But it also suffers from Vanishing Gradient Problem.
It is usually used in hidden layers of a neural network.

If we look at the derivative in the above graph, we will find that tanh function is steeper than sigmoid function. Hence, what we need out of two totally depends on the gradient required.
-
ReLU Function
The ReLU function is the Rectified Linear Units. It is the most widely used activation function.
f(x)=max(0,x)
The graphical representation of ReLU is:

It seems like the same linear function in the positive axis, but no ReLU is non-linear i.e. it can be easily used to backpropagate the errors and can have multiple layers for activation. The range of ReLU is [0 to inf).
ReLU is less computationally expensive than tanh and sigmoid functions as it involves the simple mathematical operations. It also rectifies the Vanishing Gradient Problem. Nowadays, almost all Deep learning models are using ReLU.
It does not activate all the neurons at the same time which is the biggest advantage of ReLU over other activation functions. You didn’t understand? Look here. If we look at the ReLU function, the values below zero i.e. all the negative values are considered to be zero and neuron does not get activated. This means at a particular time only a few neurons are activated and are resulting in the network to be sparse and more efficient.
Now, have a look at the gradient:

The gradient of values below zero is zero i.e. the network is not learning or the error is not backpropagated in this region which results in the Dead Neurons which then never gets activated.
-
Leaky ReLU
Leaky ReLU is a modification function. It is introduced to solve the problem of dying neurons during training of gradients in ReLU function. A small slope is introduced to keep the neuron updates alive.
The graphical representation of Leaky ReLU is:
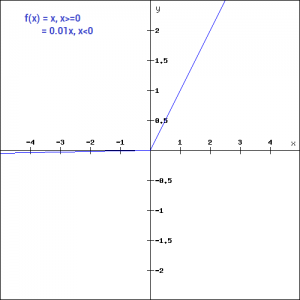
In the above graph, generally, the value of a is 0.01. If the value is not 0.01 then the function is called Randomized ReLU or Maxout function
A new variant is formed which is both ReLU and Leaky ReLU called MaxOut function.

-
Softmax
Softmax is a non-linear function. It is a type of sigmoid function but is handy while trying to handle multiple classes or classification problems.
As we know for output layer of binary classification we use the sigmoid function but for more than two types of classification, we should use softmax function.
It tries to attain the probabilities of each input of the class. The softmax function would squeeze the outputs for each class between 0 and 1 and would also divide by the sum of the outputs. It can be explained as:
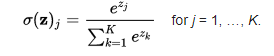
Conclusion:
Which activation function to be used?
From above, we can conclude that ReLU activation function is the best choice among all for the hidden layers. It is also a general activation function. And if we found any dead neuron case, the Leaky ReLU must be used.
We should avoid using tanh function as it produces a vanishing gradient problem which results in degradation of our model performance.
For binary classification problem, the sigmoid function is a very natural choice for the output layer and if classification is not binary then softmax function should be suggested.
In the next blog, we are going to learn about How Neural Network works? So Stay updated!
If you have any queries, you can write in the comments!
References:
[1] http://www.leadingindia.ai
[2] https://www.geeksforgeeks.org/activation-functions-neural-networks/
[3] https://towardsdatascience.com/activation-functions-and-its-types-which-is-better-a9a5310cc8f
[5] https://towardsdatascience.com/activation-functions-neural-networks-1cbd9f8d91d6
Leave a comment